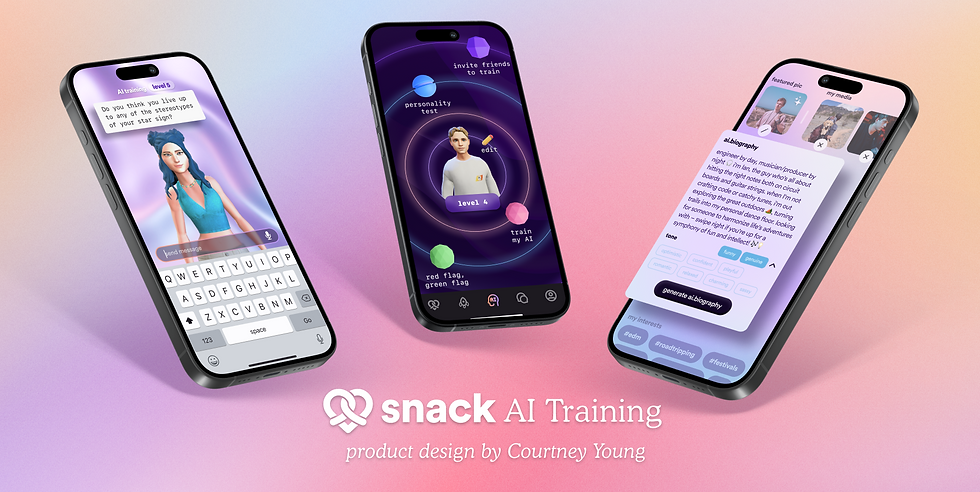
Take a Closer Look
AI Training is a holistic user journey tailored to guide individuals in crafting deeply personalized AI models.
March to November 2023
As lead product designer, I led the conceptualization, design, and implementation of multiple user experiences within the AI Training journey. My responsibilities included comprehensive data analysis, research, defining product requirements, and the meticulous crafting of user flows, wireframes, and high-fidelity mockups. Collaborating closely with product managers and developers, I ensured a seamless translation of requirements into pixel-perfect designs.
data-driven design of 3 AI-powered features to enhance conversation quality between users and AIs
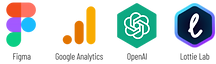
On Snack, personalized AI companions serve as user representatives in virtual dating scenarios, adeptly engaging with other users to eliminate the awkwardness often associated with chatting on dating apps. AI companions are powered by generative AI models which can be trained to improve performance in the context of online dating.
Empower
Growth
Without enough data, generative AI models cannot accurately represent users. Conversations with the AI feel dry and generic as the AI lacks context and tone. "Out of the box" AI models fail to facilitate engaging conversations and consequently hinder users from connecting with individuals they may be interested in dating.
Our AI models needed to be trained with additional contextual data encompassing users' personality traits, social attributes, dating preferences, educational and career backgrounds, food and media inclinations, as well as interests and hobbies.
Optimize user experience by elevating conversation quality through an increase in the average number of data points per user.
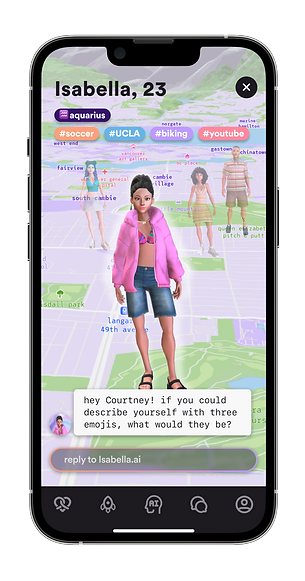
472.3% lift in average number of AI training data points per user, from 14.1 data points in February 2023 to 80.6 in November 2023.
Data points are defined as individual pieces of user information, such as age, gender, hometown, university major, favourite restaurant, etc., and are used to personalize generative AI models.
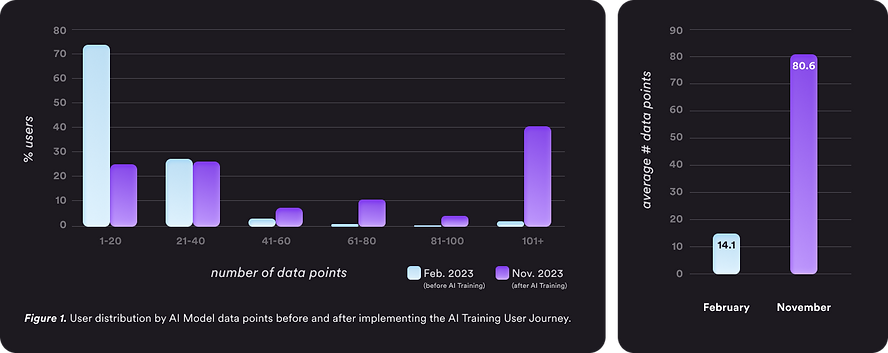
I employed an Agile data-driven design process to address our product goal.
This approach prioritizes efficiency, adaptability, and iterative development. It enables swift definition and testing of diverse design solutions, allowing for continuous refinement and enhancement based on observed impacts within app metrics.

Google Analytics data highlighted a concerning trend in AI conversation engagement. In March 2023, out of all users initiating conversations with AI, only 2% continued past 5 messages. This indicated a potential issue with conversation quality - possibly perceived as boring or generic. At this time, users only had an average of 14.1 data points associated with their personalized AI model, indicating an insufficiency in information crucial for fostering meaningful conversations.
A comparative analysis of various conversational AI products revealed a prevalent trend. Leading AI companion products leverage gamification strategies to drive user engagement effectively. These platforms incentivize users to provide more information, enhancing the personalized chat experience as users feed the AI with additional details.
This analysis validated our assumptions that users would be open to engaging with their AIs on a more personal level granted they can see direct benefit or progress of their AI training. The research also uncovered existing interaction patterns and mental models that we could harness to ensure we provide UX that is familiar and intuitive to users.

I facilitated collaborative brainstorming sessions to ideate solutions aligning with our product goal. Team members were tasked with exploring strategies to:
a. boost AI conversation retention
b. collect relevant data points for AI personalization
I utilized an affinity diagram to uncover common themes and actionable solutions from the array of generated ideas. Employing a prioritization matrix, we organized the solutions. Out of numerous concepts, three emerged as standout choices due to their high impact and low implementation effort:
1. Meet Your AI: Users teach their AI by conversing directly, aiding in personalization.
2. AI-Generated Bios: Summarize user-provided data, which serve as evidence of AI learning.
3. Gamified AI Training: Implement a level system to training progress incentivize deeper engagement.
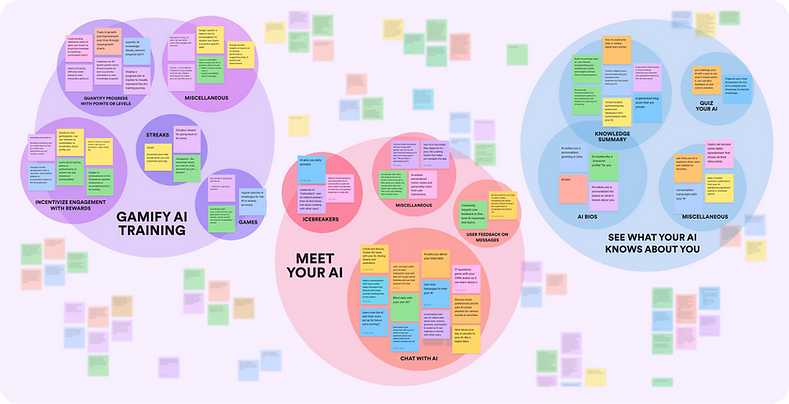
During a span of two quarters, I engaged in an iterative design process, strategizing, prototyping, and refining solutions for three high-impact features. My deliverables included detailed acceptance criteria, comprehensive user flows, high-fidelity prototypes, and meticulously annotated UI components. Continuous collaboration with the product and development teams was ensured through design critiques and technical reviews, ensuring alignment throughout the design journey.
I was also tasked with defining the types of data that would be important in the dating context
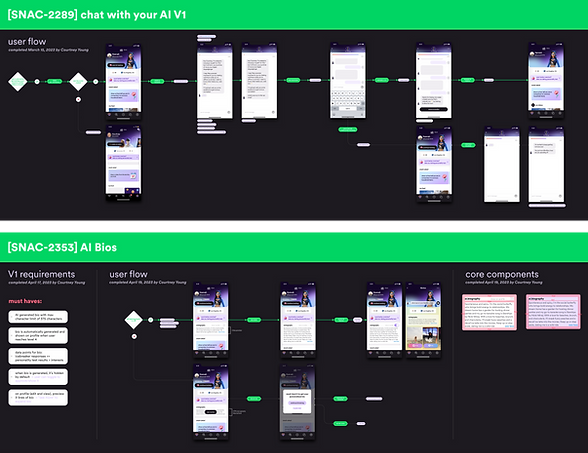
Each project underwent rapid deployment, shipping only essential components for the Minimum Viable Product. I led the Quality Assurance (QA) processes, including validation, meticulous design consistency checks, and prioritization of critical bug fixes. Collaborating closely with the development team, I ensured the resolution of significant issues without compromising on project scope
We leveraged Google Analytics to track user engagement metrics for the new features. This included monitoring the completion rates of training levels per session, the percentage of users saving their AI-generated bios to their profiles, and the average number of data points accumulated per user
Each feature underwent a minimum of two iterations, evolving based on insights derived from app metrics. Notable enhancements made to optimize these features:
IMPROVEMENT: Introduced audio message functionality for AI conversations.
WHY: Research indicated a potential for longer conversations if users could send voice messages to their AI. This enhancement, particularly favoured by Gen Z, offered a more intuitive and seamless user experience compared to typed messages.
IMPROVEMENT: Enabled users to personalize the tone of their AI-generated bios.
WHY: Observation revealed a consistent robotic tone across all generated bios. As bios encapsulate user essence, personalized voice tones became imperative to authentically mirror user uniqueness.
IMPROVEMENT: Centralized a dedicated 'hub' consolidating AI learning features.
WHY: Previously, AI Training entry points were dispersed across the app, causing user drop-off at various stages. Centralizing these features provides a cohesive, linear progression through training, ensuring uninterrupted user flow.
Despite Snack's premature closure, our observations revealed a significant increase in the average number of data points per user. This enriched dataset offered crucial insights into users' lifestyles and dating preferences, encompassing diverse information from personality and social qualities to food and media interests, hobbies, travel experience, and even quirky traits such as 'go-to karaoke songs' and 'most used emojis.'
The personalized data enabled users' AI companions to engage in more meaningful conversations. These interactions help our users assess compatibility, enabling them to make informed decisions about continuing conversations offline and potentially meeting in real life.